In this article, we will discuss how to do a one- sample proportion z-test in R with some practical examples.
What is One- Sample Proportion z-test ?
One-sample proportion z-test is used to conduct a hypothesis test about a population proportion(p). It is used to estimate the difference between the proportion of responses(or a number of successes) in a sample data and the actual proportion in the population data from which we draw the sample.
Conditions required to conduct one sample proportion z test
Assumptions for the one sampleproportion z-test are as follows:-
- The sample should be drawn at random from the population.
- Population should follow a binomial distribution.
- np0>10 & n(1-p0)>10 where n is sample size and p is the hypothesized value for the population proportion.
- The population size should be 10 times larger than the sample size.
Hypothesis for the one sample proportion z-test
Let p0 denote the hypothesized value for the proportion.
p denotes the population proportion
Null Hypothesis:
H0 : p=p0 The population proportion is equal to hypothesized proportion.
Alternative Hypothesis: Three forms of alternative hypothesis are as follows:
- Ha : p < p0 Population proportion is less than the hypothesized proportion.It is called lower tail test (left-tailed test).
- Ha : p > p0 Population proportion is greater than the hypothesized proportion.It is called Upper tail test(right-tailed test).
- Ha : p ≠ p0 Population proportion is not equal to hypothesized proportion.It is called two tail test.
Formula for the test statistic one proportion Z test is:
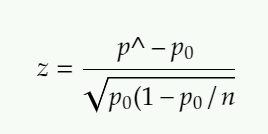
where :
n: sample size
p^: observed sample proportion
p0: hypothesized population proportion
Functions in R for one proportion z-test
To perform one proportion z-test, we will use the following functions from the R stats library.
We will use binom.test() or prop.test() function from R stats library
binom.test() :
If n ≤ 30 i.e when sample size is small then we use binom.test() function to perform one -proportion z-test.
The binom.test() function uses the following basic syntax:
binom.test(x, n, p = 0.5, alternative = c("two.sided", "less", "greater"), conf.level = 0.95)
where:
x: The number of successes
n: The number of trials.
p: The hypothesized probability of success.
alternative: The alternative hypothesis for the test.It can be ‘greater’, ‘less’, ‘two.sided’ based on the alternative hypothesis.
conf. level: confidence level of the interval
prop.test() :
If n >30 i.e when sample size is large then we use prop.test() function to perform one -proportion z-test.
The prop.test() function uses the following basic syntax:
prop.test(x, n, p = NULL, alternative = c("two.sided", "less", "greater"), conf.level = 0.95, correct = TRUE)
where:
x : The number of successes
n: The number of trials.
p: The vector of probabilities of success.
alternative: The alternative hypothesis for the test.It can be ‘greater’, ‘less’, ‘two.sided’ based on the alternative hypothesis.
conf. level: confidence level of the interval.
correct: a logical indicating whether Yates’ continuity correction should be applied or not where it is possible.
Summary for the one sample Proportion Z-test
Left-tailed Test | Right-tailed Test | Two-tailed Test | |
Null Hypothesis | H0 : p≥p0 | H0 : p≤p0 | H0 : p=p0 |
Alternate Hypothesis | Ha : p<p0 | Ha : p>p0 | Ha : p ≠ p0 |
Test Statistic | z= (p^ – p0)/√(p0(1- p0 )/n | z= (p^ – p0)/√(p0(1- p0 )/n | z= (p^ – p0)/√(p0(1- p0 )/n |
Decision Rule: p-value approach (where α is level of significance) | If p-value ≤α then Reject H0 | If p-value ≤α then Reject H0 | If p-value ≤α then Reject H0 |
Decision Rule: Critical-value approach | If z ≤ -zα then Reject H0 | If z ≥ zα then Reject H0 | If z ≤ -zα/2 or z ≥ zα/2 then Reject H0 |
How to do one proportion z-test in R?
We will calculate the test statistic by using one proportion z-test.
Procedure to perform One Proportion Z-Test in R
Step 1: Define the Null Hypothesis and Alternate Hypothesis.
Step 2: Decide the level of significance α (alpha).
Step 3: Calculate the test statistic using the binom.test() or prop.test() depending upon the sample size.
Step 4: Interpret the one-proportion z-test results.
Step 5: Determine the rejection criteria for the given confidence level and conclude the results whether the test statistic lies in the rejection region or non-rejection region.
Let’s see practical examples that show how to use the binom.test() or prop.test() function in R.
Examples of One Proportion z-test in R
Example 1: Right-tailed one proportion test in R
An auditor for the Online Service wants to examine its special two-hour priority order delivery to determine the proportion of the orders that actually arrive within the promised two-hour period. A randomly selected sample of 1500 such orders is found to contain 1150 that were delivered on time. Does the sample data provide evidence to conclude that the percentage of on-time orders is more than 75%? Test at 5% level of significance.
Solution: Given data :
sample size (n) = 1500
number of success (x) = 1150
sample proportion (p^) = x/n = 1150/1500 = 0.7666
hypothesized population proportion (p0) = 0.75
level of significance (α) = 0.05
confidence level = 0.95
Let’s solve this example by the step-by-step procedure.
Step 1: Define the Null Hypothesis and Alternate Hypothesis.
Let p0 denote the hypothesized value for the proportion.
p denotes the population proportion
Null Hypothesis: The population proportion is equal to 0.75 (i.e. 75%).
H0 : p= 0.75 (right-tailed test)
Alternate Hypothesis: The population proportion is greater than 0.75 (i.e. 75%).
Ha : p > 0.75
Step 2: level of significance (α) = 0.05
Step 3: Calculate the test statistic using the below code.
Since here sample size = 1500 >30.
So we use prop.test() in this example.
# Perform one-proportion z-test prop.test(x=1150, n=1500, p=0.75, alternative="greater")
Specify the alternative hypothesis as “greater” because we are performing a right-tailed test. The results for the one-proportion z-test are as follows.
#Results 1-sample proportions test with continuity correction data: 1150 out of 1500, null probability 0.75 X-squared = 2.1342, df = 1, p-value = 0.07202 alternative hypothesis: true p is greater than 0.75 95 percent confidence interval: 0.7478919 1.0000000 sample estimates: p 0.7666667
Step 4: Interpret the one-proportion test results.
How to interpret one proportion z-test results in R?
Let’s see the interpretation of one-proportion z-test results in R.
data: This gives information about the data used in the one-proportion z-test.
X-squared: the value of Pearson’s chi-squared test statistic.
df: the degree of freedom of the approximate chi-squared distribution of the test statistic.
p-value: This is the p-value corresponding to a statistic. In our case, the p-value is 0.07202.
alternative: It is the alternative hypothesis used for the z-test. In our case, an alternative hypothesis is population proportion is greater than 0.75 i.e. right-tailed.
95 percent confidence interval: This gives us a 95% confidence interval for the true proportion. Here the 95% confidence interval is [0.7478919,1.0000000].
sample estimates: It gives the sample proportion. In our case, the sample proportion is 0.7666667.
Step 5: Determine the rejection criteria for the given confidence level and conclude the results whether the test statistic lies in the rejection region or non-rejection region.
Conclusion:
Since the p-value[ 0.07202 ] is greater than the level of significance (α) = 0.05, we fail to reject the null hypothesis.
This means we have sufficient evidence to say that the population proportion is equal to 0.75.
Example 2: Two-tailed one proportion test in R
In a sample of 20 students in College,11 are tea-drinkers and the rest are coffee-drinkers. Can we assume that both tea and coffee drinkers are equally popular in college at the 5% level of significance?
Solution: Given data :
sample size (n) = 20
Number of tea-drinkers (x)= 11
sample proportion (p^) = number of tea-drinkers /sample size
p^ = 11/20 = 0.55
Let’s solve this example by the step-by-step procedure.
Step 1: Define the Null Hypothesis and Alternate Hypothesis.
let p be the population proportion for the tea drinkers.
Null Hypothesis: Both tea and coffee drinkers are equally popular in the college
H0 : p = 0.5
Alternate Hypothesis: Tea and Coffee drinkers are not equal in college.
Ha : p ≠ 0.5
Step 2: level of significance (α) = 0.05
Step 3: Calculate the test statistic using the below code.
Since here, sample size = 20 <30.
So we use binom.test() in this example.
# Perform one-proportion z-test binom.test(x=11,n=20,p=0.5,alternative = "two.sided")
Specify the alternative hypothesis as “two.sided” because we are performing a two-tailed test. The results for the one-proportion z-test are as follows.
#Results Exact binomial test data: 11 and 20 number of successes = 11, number of trials = 20, p-value = 0.8238 alternative hypothesis: true probability of success is not equal to 0.5 95 percent confidence interval: 0.3152781 0.7694221 sample estimates: probability of success 0.55
Step 4: Interpret the one-proportion test results.
How to interpret one proportion z-test results in R?
Let’s see the interpretation of one-proportion z-test results in R.
data: This gives information about the data used in the one-proportion z-test. It tells the number of successes and the number of trials.
p-value: This is the p-value corresponding to a statistic. In our case, the p-value is 0.8238.
alternative: It is the alternative hypothesis used for the z-test. In our case, an alternative hypothesis is a Tea and Coffee drinkers are not equal in college, i.e. two-tailed.
95 percent confidence interval: This gives us a 95% confidence interval for the true proportion. Here the 95% confidence interval is [0.3152781,0.7694221].
sample estimates: It gives the probability of success. In our case, the probability of success is 0.55.
Step 5: Determine the rejection criteria for the given confidence level and conclude the results whether the test statistic lies in the rejection region or non-rejection region.
Conclusion:
Since the p-value[0.8238] is greater than the level of significance (α) = 0.05, we fail to reject the null hypothesis.
This means we have sufficient evidence to say that tea and coffee drinkers are equally popular in college.
The R Stats Package is needed to do a z-test in R.
Summary
I hope you found the above article on One Proportion z-test in R with Examples informative and educational.